Project Details
BloomiX: Transforming Fertility Care with AI-powered Sperm Analysis and Selection
- Future with AI
- Future with AI
- Future with AI
- Future with AI
- Future with AI
- Future with AI
- Future with AI
- Future with AI
- Future with AI
- Future with AI
- Future with AI
- Future with AI
- Future with AI
- Future with AI
- Future with AI
- Future with AI
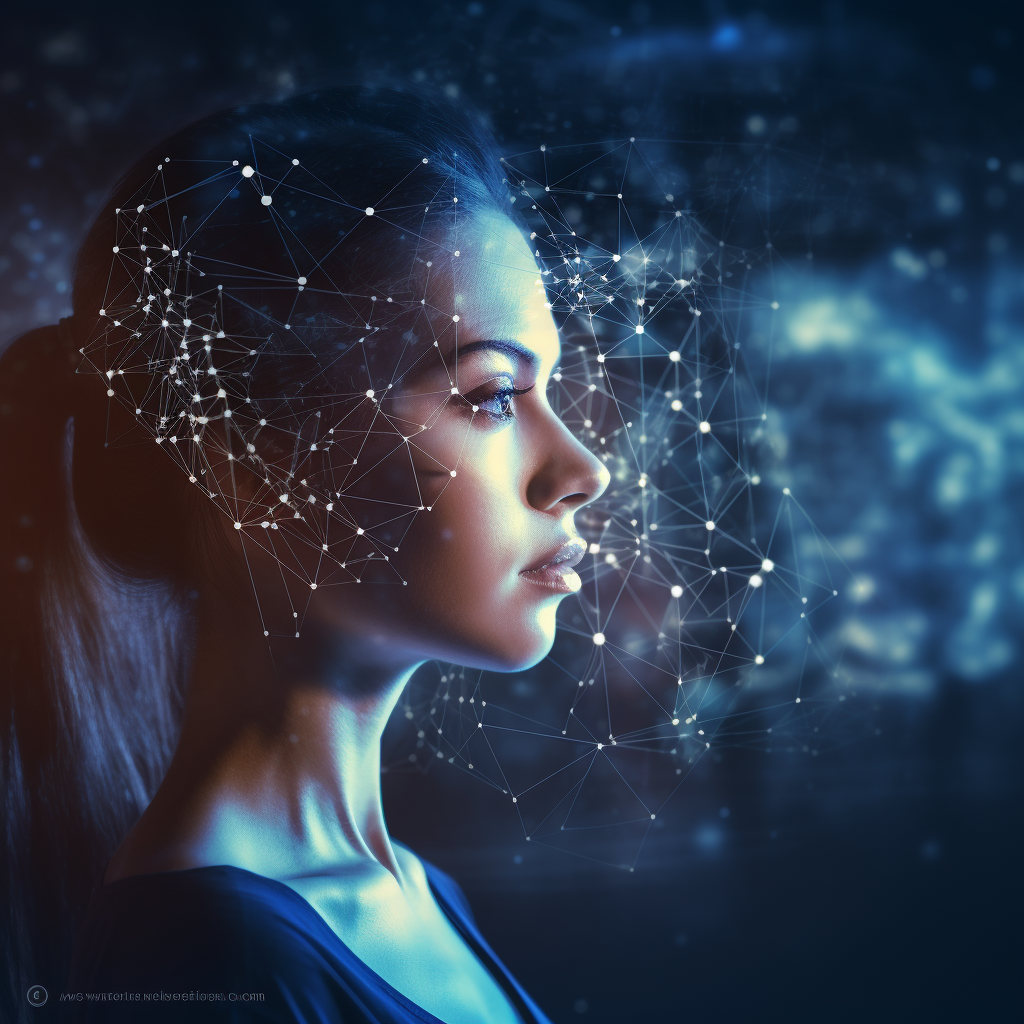
Competitive Advantages
Advanced AI Technology
High-Quality Datasets
Multi-Faceted Sperm Analysis
Real-Time Assistance
Improved Outcomes
Our Company Brochure
BloomiX redefines fertility care with Artificial Intelligence, advancing the science of sperm analysis, and making dreams come true.
Deep Learning for Sperm Detection
BloomiX is a comprehensive deep learning-based model for sperm analysis and selection that combines various algorithms and models to provide accurate and reliable results. The model is designed to work with both still images and live video, making it suitable for both laboratory and clinical settings. It uses a range of deep learning models, including Convolutional Neural Network (CNN), Recurrent Neural Network (RNN), U-Net, and YOLO, to detect and analyze sperm abnormalities, track sperm motility, and predict sperm morphology. In addition to these features, the system also offers real-time analysis,
data visualization, and reporting capabilities.
Project Overview:
Our primary objective is to develop a clinical decision support system (CDSS) that assists embryologists in selecting the most viable sperm for use in in vitro fertilization (IVF) and
intracytoplasmic sperm injection (ICSI) procedures.
Project Specification:
- The system should suggest appropriate normal sperm to embryologists based on sperm morphology, motility, and DNA integrity
- The system should assist embryologists in detecting the most prominent sperm in real-time microscopic live video
- The system should be able to analyze non-stained sperm images and videos
- The system should provide accurate results even on low-magnification cameras.
Key Features:
Deep Learning Models: Our system includes a range of deep learning models, including CNN, RNN, U-Net, and YOLO, to provide accurate and reliable results for sperm analysis and selection.
Real-Time Analysis: Our system provides real-time analysis and visualization of sperm samples, allowing users to quickly and easily identify abnormalities, track sperm motility, and select normal sperm samples.
User Interface: Our system includes a user-friendly interface that allows users to easily navigate and interact with the system. The interface includes features such as live video display, region of interest selection, and heatmap visualization.
Scalability: Our system is designed to be scalable, allowing it to handle large volumes of data and work with multiple users simultaneously.
Versatility: Our system is designed to work with both still images and live video, making it suitable for both laboratory and clinical settings. It also includes an optional feature for checking sperm DNA integrity, providing additional value for
users.
Discovery Phase
Data Preparation
Model Development
Algorithms and Models:
In order to achieve the “Predict Sperm” use case scenario, several machine learning algorithms and models are used in the system. These include:
U-Net and YOLO for Object Detection:
The U-Net is a CNN architecture used for image segmentation tasks. It is used in this system for detecting and localizing the sperm in the live video feed, by creating a bounding box around the selected sperm. Additionally, YOLO (You Only Look Once) is another object detection model used in the system to detect the sperm in the images. Both models help to identify the exact location of the sperm within the image, enabling the system to isolate it and analyze it more accurately.
Convolution Neural Network for sperm head abnormality detection:
ACNN is used for analyzing the region of interest, which is the sliced image of the selected sperm from the original image. This model is used to determine whether the selected sperm’s head morphology is normal or abnormal. The CNN model is trained on the MHSMA dataset, which contains labeled sperm images with normal and abnormal sperm head morphology.
Recurrent Neural Network for sperm tracking and sperm motility detection:
A recurrent neural network (RNN) is used for tracking and analyzing the motility of the sperm. The RNN model is trained on a dataset containing labeled sperm tracks and corresponding motility scores. The model analyzes the movement patterns of the sperm and predicts a motility score, which indicates the degree of mobility and fertility of the sperm.
Main Services
the combination of these algorithms and models allows the system to accurately predict sperm head morphology, track sperm movement, and analyze sperm motility in real-time.
Our system uses Convolutional Neural Network (CNN) to detect sperm head and sperm head vacuole abnormalities with high accuracy. The CNN model has been trained on a large dataset of human sperm images, labeled by experts for normal and abnormal sperm acrosome, head, vacuole, and tail. The system provides real-time predictions and visualizations, allowing users to quickly and easily identify abnormal sperm samples.
Our system uses Recurrent Neural Network (RNN) to track sperm movement and detect sperm motility. The RNN model has been trained on a dataset of sperm videos, allowing the system to accurately track sperm movement and identify motility patterns. The system also provides real-time analysis and visualization of sperm motility data.
Our system allows users to select normal sperm samples from still images and live video using a combination of YOLO and U-Net models. The YOLO model is used to detect sperm samples in video, while the U-Net model is used to segment the sperm head from the background. This allows users to quickly and easily identify and select normal sperm samples for further analysis.
Our system includes an optional feature for checking sperm DNA integrity. This feature uses a combination of CNN and RNN models to analyze sperm images and videos for DNA fragmentation, allowing users to identify potential DNA damage in sperm samples.
Revolutionary AI-Based Features for Enhanced Fertility Care
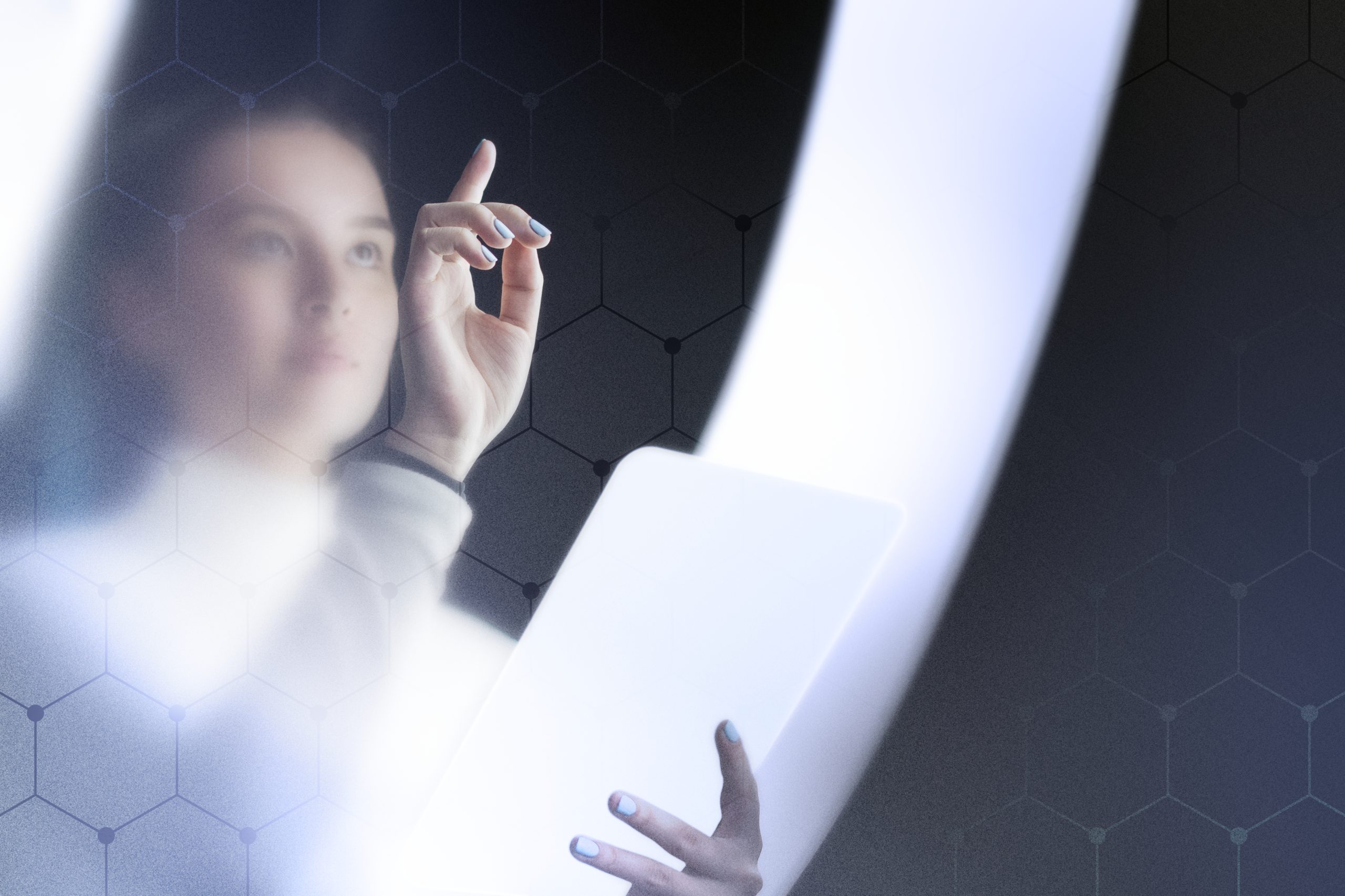
Real-Time Sperm Analysis
Instantly assess sperm morphology, motility, and DNA integrity in live videos
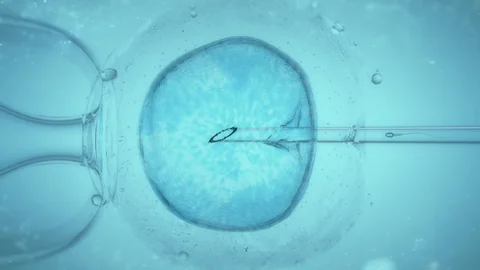
Expert-Guided Selection
Embryologists can select and analyze specific sperm for personalized assessments
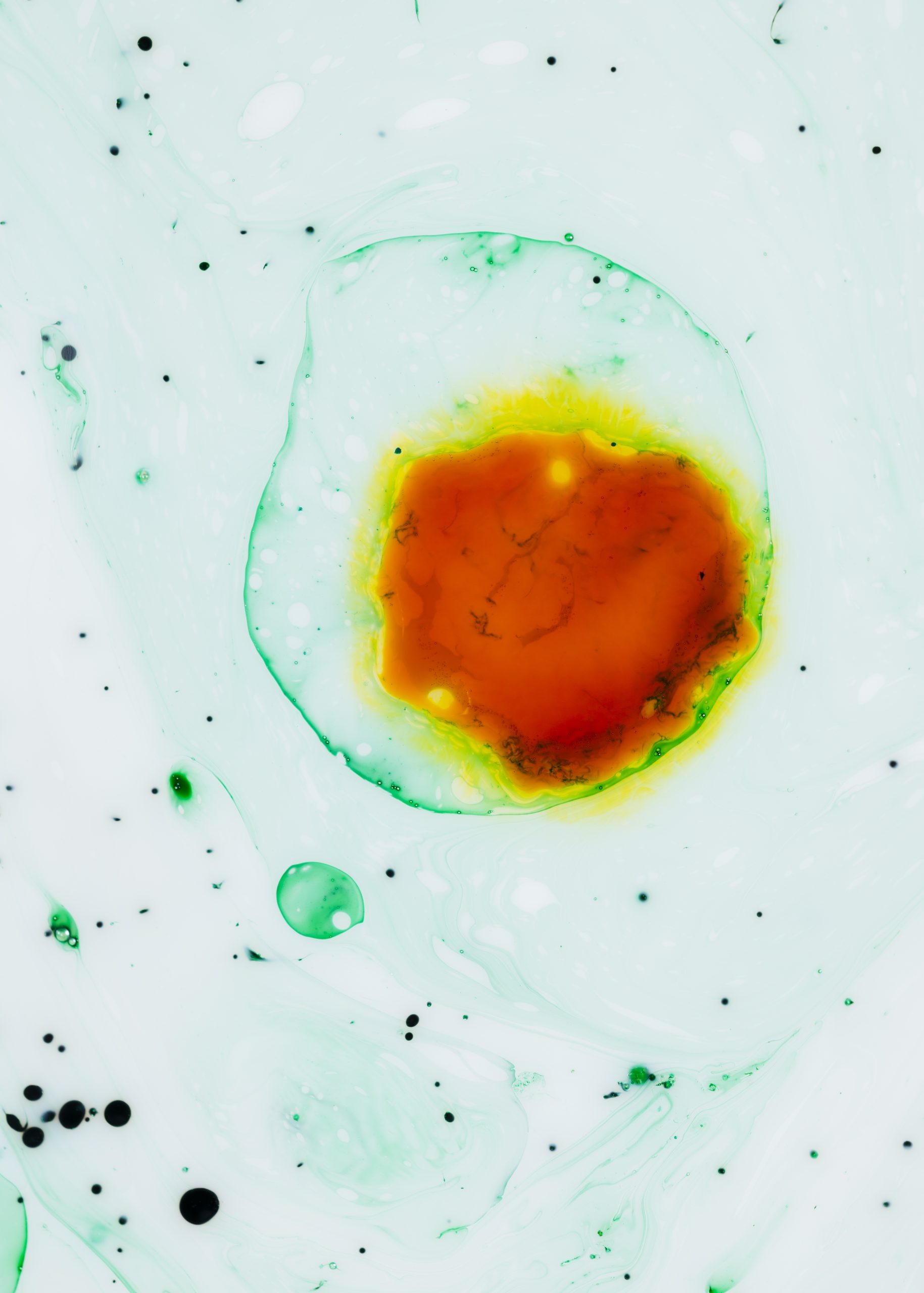
Explainable AI
Gain insights into AI decisions through heatmaps, increasing transparency and trust
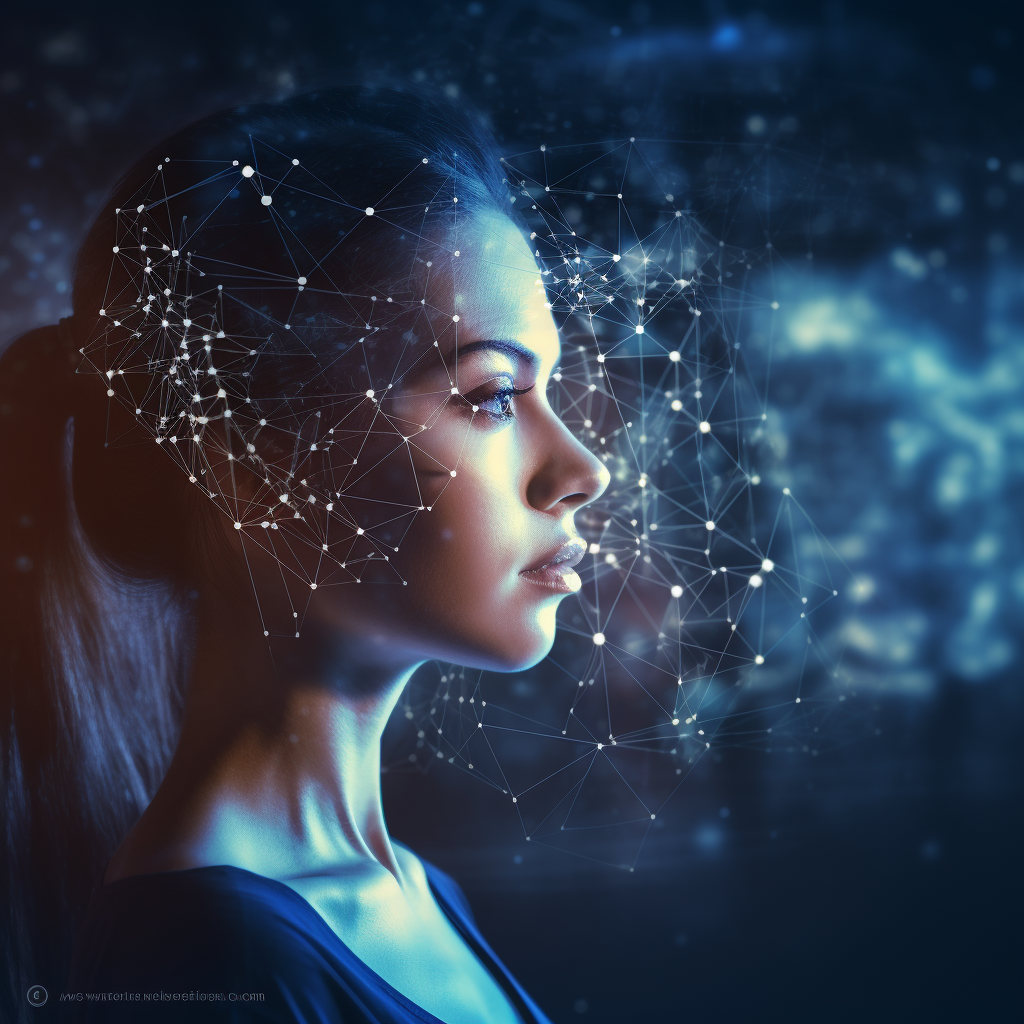
Continuous Learning
AI models improve over time, adapting to new data and advancing accuracy